Updated June 1, 2024: I have modified and updated this post in response to an email I received from Greg Lindahl, CTO of Common Crawl. The complete post history can be found here.
Mozilla recently published an excellent new report about Common Crawl, the non-profit whose web crawls have played an important role in the development of numerous large language models (LLMs). Written by Stefan Baack and Mozilla Insights, the report is based on both public documents and new interviews with Common Crawl’s current director and crawl engineer, and goes into some detail about the history of the organization, and how its data is being used.
As I have written elsewhere, Common Crawl occupies a unique place in the LLM ecosystem. Although there are plenty of LLMs that have been trained using other data sources (including GPT-2, for which the creators directly scraped web pages linked from reddit), numerous landmark LLMs have used at least some Common Crawl data for pretraining.
The advantages of using Common Crawl for this purpose are obvious—it is huge and broad, comprising many terabytes of text content scraped from the web.1 As the name implies, this is a web crawl—starting from a few billions of seeds, the crawlers follow links and save content as they go.2 Common Crawl’s focus is on collecting the raw HTML for web pages, rather than things like images or CSS files. This makes it very different from a more comprehensive web archive, such as the Internet Archive, which strives to maintain greater provenance, especially in the context of their Wayback Machine. Using that service, it is possible to view and even interact with archived versions of web pages, similarly to how they would have appeared and functioned at many different points in the past.3
As detailed in the Mozilla report, Common Crawl was created in 2007 as a non-profit to provide large scale data, copied from the web, for purposes in research, business, and education. The data they collect is made freely available in snapshots, distributed via Amazon Web Services (AWS). The organization has, by now, had an enormous impact, having been cited by over 10,000 research papers, but initially it was not clear what this was all going to lead to.
The first NLP paper I am aware of that used this data was a 2013 Machine Translation paper by Philipp Koehn, Chris Callison-Burch, Adam Lopez, and others, called “Dirt Cheap Web-Scale Parallel Text from the Common Crawl”.4 To help locate this work in time, it seems like a large chunk of the parallel language text that those authors were able to extract from Common Crawl came from hotel booking sites.
Coincidentally enough, the first LLM trained on CommonCrawl data (that I am aware of) was Grover (published in 2019), a model trained to generate (and defend against) fake news, developed by a team led by Rowan Zellers and Ari Holtzman, both of whom I shared an office with while at the University of Washington.5 This was then followed by RoBERTa, T5, and GPT-3. In addition, archived snapshots of Common Crawl have been preserved in datasets such as C4 and The Pile, which in turn have now been used to train many other models, such as LLaMa.
Going farther back in time, the Wayback Machine’s first snapshot of the Common Crawl website is from March 28, 2008. At that point, it was pretty barebones, just providing the organization’s mission at the time: “CommonCrawl’s mission is to build, maintain and make widely available a comprehensive crawl of the Internet for the purpose of enabling a new wave of innovation, education and research”. There was also an FAQ, aimed largely at people who might be noticing Common Crawl’s bot visiting their websites, and wondering what it was.
By April 19, 2009, Common Crawl’s website had added a beta rollout of a URL search feature. By October 20, 2011, they had a new website, and were finally providing actual web data, distributed through a requester-pays API on AWS. The corresponding terms of use suggested “Don’t break the law or do anything illegal with our site or data”, and listed “Violate other people’s rights (IP, proprietary, etc.)” and “Invade other people’s privacy” as examples of “illegal stuff you can’t do”.
The new report from Mozilla does an especially good job of trying to correct a few key misconceptions. First among these is the idea that Common Crawl somehow represents “the entire internet”. Far from this being the case, the web dumps provided by Common Crawl only index and distribute a small fraction of what is technically available.
Surprisingly, despite the title of their report (“Training Data for the Price of a Sandwich”), the one area that the Mozilla authors do not discuss in detail is how Common Crawl was actually financed. They mostly note in passing that, although Common Crawl is now seeking additional funding in the form of donations, it has been primarily funded since the beginning by its original founder, Gil Elbaz; but that’s about it.
What this misses is just how much money Elbaz has poured into the enterprise. Before founding Common Crawl, Elbaz was a co-founder of a start up called Applied Semantics, which was acquired by Google in 2003 for $102 million, along with the company’s AdSense technology. In 2007, Elbaz left Google to start Factual, (eventually acquired by Foursquare), with the intention of creating an open dataset to make sure the search giant didn’t have a total monopoly on large scale internet data.6 In the same year, he also created the Common Crawl foundation.7 As Common Crawl’s current mission states, it aims to provide “high quality crawl data that was previously only available to large search engine corporations.”
Using the tax filings data available from ProPublica, we can see that Elbaz has donated, through his foundation, around 4 million dollars to Common Crawl over the decade and a half from 2007 through 2022. An additional $155,000 was donated by Microsoft (in two chunks) about midway through that period. That, however, is the total sum of contributions that the non-profit has received up through 2022, which is the latest year for which data is currently available.8
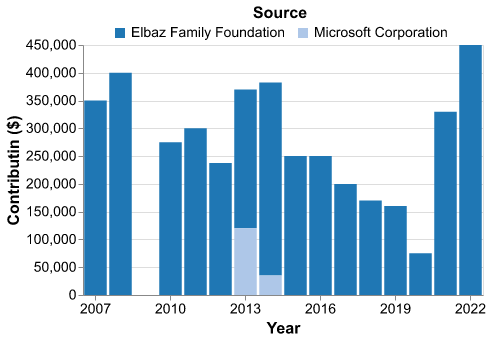
Common Crawl Funding (2007-2022).
On the one hand, four million dollars is a remarkably small amount of money, considering the impact that Common Crawl has had. It is becoming routine to hear about engineers working for OpenAI with high six- or even seven-figure salaries, and single LLM training runs now sometimes cost in the tens (or even hundreds) of millions of dollars, just to cover the cost of computation. From that perspective, $4 million seems like a drop in the bucket for a piece of infrastructure that has become such an important part of the LLM ecosystem.
On the other hand, $4 million is also a lot of money for an individual to invest in what might have seemed, especially early on, like something of a passion project. Much like Brewster Kahle, creator of the Internet Archive, and another tech entrepreneur turned non-profit founder, Elbaz chose to take the earnings from the sale of his early ventures, and apply them to something somewhat singular and quirky.
Interestingly, while Common Crawl is the largest item on the balance sheet of the Elbaz Family Foundation, the foundation has also donated almost as much money to the X-Prize Foundation. Over the years it has also contributed generously to CalTech (Elbaz’s alma mater), Claremont McKenna College, the UCLA law school, and many other recipients, including dozens of primarily liberal causes, such as the Natural Resources Defense Council, the Earth Island Institute, the Anti-Defamation League, and Iridescent Learning.
In some ways, Common Crawl has succeeded wildly in its mission, and for an incredibly economical price. There is no doubt that it has contributed massively (if indirectly) to “a new wave of innovation, education and research”, and it seems to (still) be almost the only openly available resource people think of for obtaining high quality crawl data at scale.
At the same time, it does seem like the primary direct beneficiaries of this work may end up being those companies, such as Google, Microsoft, OpenAI, and Meta, that have leveraged the existence of Common Crawl data in the process of inventing a new type of knowledge infrastructure—one which they seem increasingly likely to own and control, at least in its most widely used forms.
In his email to me, Greg Lindahl argued that “scraping” is not the right word for what Common Crawl does. To me, it still seems like the appropriate term, as per the definition of “copy (data) from a website using a computer program” (Oxford Languages). However, I do acknowledge Greg’s point that it may have a negative connotation, especially to publishers and webmasters. To be clear, I am using “scraped” in a strictly neutral sense, that does not necessarily imply anything illegal or inappropriate, just the act of copying data from a website. It is also important to note that, as per the Mozilla report, Common Crawl strives to act within the limits of US fair use doctrine, and, for example, avoids making complete copies of any single web domain. ↩︎
Greg also very helpfully pointed out that no web crawls would start from only a few seeds; he explained that Common Crawl was originally bootstrapped from a donation of over a billion URLs from the search engine blekko, where Greg was previously a co-founder and CTO. ↩︎
My original post unintentionally implied that Common Crawl is not an archive at all, which Greg challenged me on. As he rightly points out, Common Crawl is just as much of an archive as the Internet Archive is, just one that happens to have different goals. I take the point, though I still think it is interesting to think through the differences between the two, especially in light of ideas from archival theory. Obviously there are major differences in affordances. But even beyond that, while there is no question that the archives made freely available by Common Crawl have enormous value, I think it is fair to say that it might not be possible to appreciate the historical importance of at least some web pages without the elements that are not saved by Common Crawl (including images and styling); in this sense, the Internet Archive still has a special and distinct importance, especially for historians. ↩︎
Interestingly, the paper’s first author, Jason R. Smith, seems to have not published much else afterwards. DBLP associates him (as Jason Smith) with another machine translation paper from Google three years later. He is listed on Jason Eisner’s webpage, (but not as Jason’s dissertation advisor), and he does not appear on Philipp’s or Adam’s web pages. My guess is that he either left or graduated and went to work for Google. ↩︎
Rowan now works for OpenAI, and Ari is starting as an Assistant Professor at the University of Chicago this fall. ↩︎
“Gil Elbaz On Google Acquiring His Company And Turning It Into A $15 Billion Business”. DealMakers: Entrepreneur | Startups | Venture Capital. https://alejandrocremades.com/gil-elbaz-on-google-acquiring-his-company-and-turning-it-into-a-15-billion-business/ ↩︎
In his email, Greg informed me that Common Crawl’s funding profile has changed dramatically since 2022, which will be reflected in the 2023 tax filings, once they are available. ↩︎